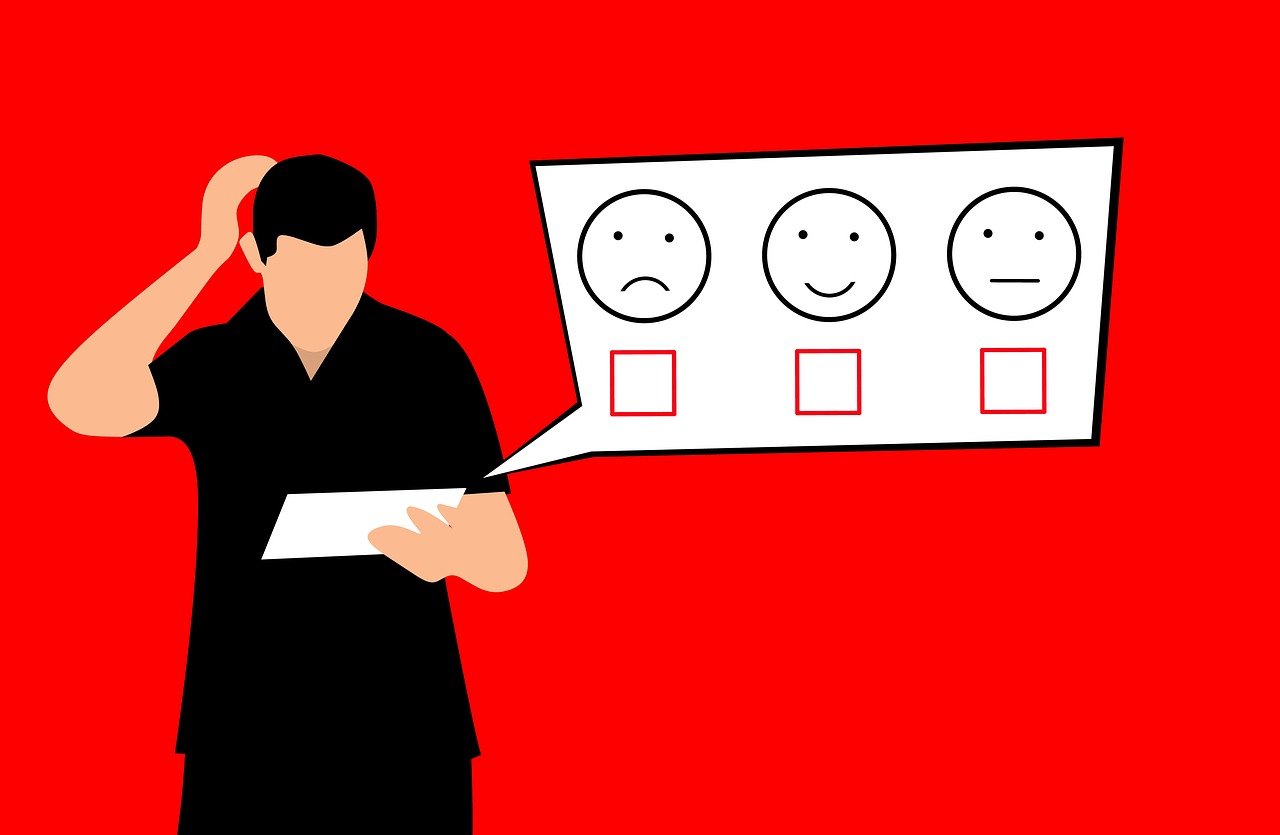
by David Campbell, VP of Product Marketing at SugarCRM
Businesses strive to create reliable, predictable, and objective processes to improve performance. Yet for many companies, there are crucial business tasks that remain more art than science. In the marketing realm, one of these persistently artistic processes is lead scoring.
Marketers understand the time-honored method of traditional lead scoring. The process calculates point scores for marketing leads based on specified attributes and activities of the leads. Points are assigned to relevant attributes, such as demographics or firmographics, as well as to activities, such as website behavior, social media actions, and campaign engagement. And each lead’s points are totaled into a composite lead score that grades the qualification of a lead for conversion to a sales opportunity.
Traditional lead scoring attempts to be data-driven, but ultimately fails because its dependencies inherently make the methodology subjective. After all, it’s up to the person defining the scoring model to ensure that the most relevant lead attributes and activities are selected for points assignment, assign the proper number of points to the attributes and activities, use historical data to drive these selections, and improve the model over time through A/B testing, campaign learnings, and incorporation of any new data.
Creating and maintaining a good lead scoring model is an art that’s very much dependent on the marketer’s skills, experience, instincts, and good fortune.
Often, traditional lead scoring models are not quantitatively informed by historical data. This is because collecting and processing the data may be impossible or prohibitively difficult. As a result, the scoring model is based on the marketer’s beliefs or interpretations of historical performance. In these instances, which are very common, the model becomes even more based on gut-feel and instinct. And many models are one-and-done; they go through very little, if any, refinement over time.
Imagine if there was a way to: Take all the information that a company had about its past, present, and prospective leads, and their marketing-relevant behaviors. Augment that information with externally sourced data about those same leads to broaden the perspective and fill in data gaps. Sift through all that data to find the lead attributes and activities that had measurable impact on historical lead conversion – not the things we think made a difference, but the things we know made a difference because the data proves it.
Based on the impact of those attributes and activities to conversion, we could then assign a weighted score to identify the leads that have the best match to historical leads that successfully converted, and then continuously update the scoring model so that scored leads always reflected the latest data and learnings. This is what predictive lead scoring does – automatically.
Predictive lead scoring uses internal lead and marketing engagement data, augmented with externally sourced company and contact data. It uses AI to learn about what really matters in converting a lead, to analyze how much it matters, and to continuously improve the model. And finally, it creates and maintains an internal scoring model that is truly data-backed and continuously improving.
Predictive lead scoring puts technology to the task of doing everything that technology does best to surface insights to empower marketers. This is a rallying cry for us at SugarCRM: Let the platform do the work to eliminate blind spots, busy work, and roadblocks.
With a data-backed rating of each prospect’s probability of converting, marketers can understand the best opportunities to focus on, and from there, everything gets easier. With greater predictability, performance improves on many levels. Organizations can increase conversions, revenues and win rates, and reduce the time and energy to optimize lead scoring models.
What’s not to like, right?
There is some understandable ambivalence with marketers adopting this technology. Some feel as if they don’t necessarily want to “let go of the wheel.” But it’s not a “black box,” – there’s progressive disclosure about how the score was arrived at, so marketers are able to understand and feel comfortable about how the technology is working.
The beautiful thing for marketers is that they don’t have to choose between lead scoring art and science; it doesn’t have to be an either/or proposition. Predictive lead scoring can be used as a complement to traditional lead scoring. Marketing teams benefit from having both human-driven and data-driven perspectives. Human-driven lead scoring presents the opportunity to incorporate scoring elements outside of AI awareness because it isn’t represented in the data. Conversely, machine-driven models can show marketers where their scoring models may be deficient and can help improve marketers’ skills in qualifying leads.
It’s an exciting time to be a marketer. Predictive lead scoring is a game-changer, helping marketers to work smarter not harder. By adding a data-driven approach, marketers can truly be free to work their craft, putting their full art and heart into it.
SugarCRM‘s Vice President of Product Marketing David Campbell leads a critical area of the Sugar team. With over 30 years of experience in software and technology and substantive achievements in all facets of product marketing and management, David has successfully conceived, developed, launched and campaigned multiple, industry-leading products and services in a variety of markets.